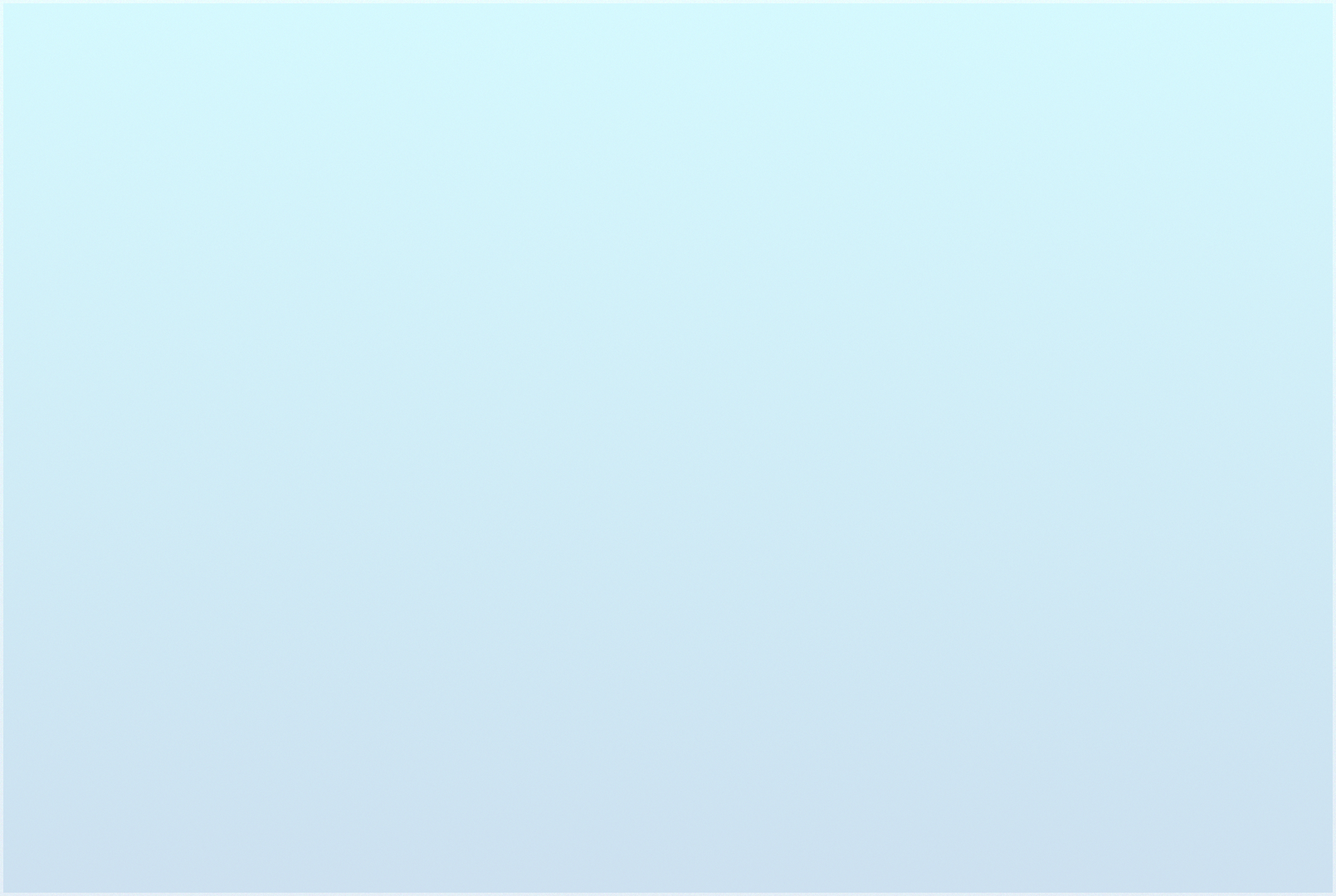
AI-Driven Deal Sourcing at the AnBridge M&A Platform
A fintech startup, Dotpoint, created the Anbridge M&A platform to streamline deal sourcing for M&A advisors. Traditionally, advisors rely on manual and inefficient processes, leading to mismatched deals and low customer satisfaction. To solve this, I designed a solution with smart buyer-seller matching and data-driven insights, enabling advisors to make faster, smarter decisions.
My Role
Product Designer
Team
1 Product Manager 1 Product Designer 2 Software Engineers 1 Business Analyst
Timeline
Sep 2024 - Jan 2025
Dot Point is a Fintech startup transforming Merge & Acquisition with AI-driven automation, offering two innovative products:
Dot Point: A proprietary trading firm specializing in APAC retail trading. It provides capital funding and strategic support, helping traders optimize and streamline financial transactions.
AnBridge: An AI-powered M&A platform designed to accelerate deal sourcing and execution for SMEs in China. It empowers M&A advisors with AI-driven deal matching and faster, more scalable transaction execution.
The Abridge platform taps into a $230 billion SME M&A market, aiming to address the needs of 315K SMEs with retiring owners seeking succession solutions.
Opportunities: How might we leverage AI to streamline M&A deal sourcing and enhance seller-buyer matching accuracy?
Mergers and acquisitions (M&A) enable companies to expand, acquire new technology, and enhance efficiency. M&A advisors play a critical role in connecting sellers with the right buyers, managing financial, informational, and strategic exchanges to facilitate successful deals.
However, traditional deal sourcing is time-consuming and prone to mismatches, leading to inefficiencies in buyer-seller connections. AI-powered matching can revolutionize this process by analyzing vast datasets, improving accuracy, reducing manual effort, and accelerating deal closures—ultimately enhancing advisor credibility and buyer satisfaction.
To better understand the challenges in M&A deal sourcing, I conducted five structured interviews with three M&A advisors and two buyers. Through open-ended discussions, I explored their deal sourcing workflows, matching processes, and decision-making challenges. I mapped their workflows, identified inefficiencies like manual processes, fragmented tools, and mismatched buyers, and synthesized key findings. These insights highlighted the need for AI-driven automation to improve matching accuracy, streamline workflows, and accelerate deal success.
M&A Advisors & SME Deal Sourcing Workflow
Before: Traditional Deal Sourcing Challenges
Previously, M&A advisors manually searched through hundreds of buyer profiles to find the right match for a seller company, often facing inefficiencies and frequent rejections.
1. Manual Data Gathering: advisors sort through massive buyer data, making the process slow and inefficient.
2. High Mismatch Rate: advisors match buyers based on personal experience, leading to 70% mismatched deals.
3. Slow Buyer Shortlisting: Manually ranking buyers is time-consuming, often leads to misaligned priorities
4. Ineffective Outreach: sending mismatched teasers lead to low buyer engagement and credibility loss.
After: AI-Power Deal Sourcing Opportunities
AI-driven deal sourcing provides advisors with instant access to a structured and high-match buyer list, making targeted outreach easier and more efficient.
1. Efficient Data Gathering - Convenient access to a vast seller network and structured buyer profiles across multiple industries.
2. Smart Matching: AI instantly recommends top buyers based on deal criteria, using matching scores and deep analytics to ensure precision.
3. Strategic Buyer Shortlisting: Advisors can compare, filter, and prioritize buyers in real time, ensuring the best-fit deals with data-backed decisions.
4. Effective Outreach – AI helps advisors contact highly-matched buyers in one click, sending customized teasers and improving buyer engagement.
Designing the AI-powered Deal Sourcing Solution
I designed the AI-powered matching solution that automates buyer recommendations, centralizes data, and enhances accuracy with customized criteria. The Matching Analysis tool provides in-depth insights, helping users evaluate various buyers, understand matching rationale, and make data-driven decisions.
1. Set customizable seller criteria for targeted seller client
2. AI recommends buyers, users shortlist top matches.
3. Assess buyer company Info and Investment criteria
4. Evaluate matching analysis and get detailed insights
Ideation & Iteration: AI Matched Buyers
To create an efficient buyer-seller matching experience, I explored four design iterations to balance clarity, insights, and decision-making. Advisors needed both quick scanning and in-depth analysis. The challenge was structuring rankings and insights for easy comparison, prioritization, and action—without overwhelming users or adding unnecessary navigation.
Version 1: Structured Table View with Buyer Match List
The first version offers a clear, scannable layout that allowed users to quickly review buyer matches. Additionally, it included quick chart insights for match distribution. However, as the amount of data increased, the interface became cluttered, making it difficult to view contextual seller information.
Version 2: Visualized Table View with Regional Match Segmentation
The second version visually groups buyers into high, medium, and low match categories. This design made decision-making more intuitive by allowing users to prioritize matches based on investment potential. However, the grouping method lacked flexibility, sometimes missing nuanced details and limiting user control over filtering criteria.
Version 3: Full-Page Card Layout for matched Buyer Profiles
The third version provides a detailed, focused display of each buyer's profile. This version worked well for deep analysis of individual buyers, but it was less efficient for high-level scanning and prioritization, making it difficult for advisors to compare multiple matches at once.
Version 4: Interactive Card View with Slide-In Panel
This version integrates a slide-in panel, enabling users to seamlessly access detailed buyer insights while keeping seller context visible at all times. The design improves decision-making efficiency by allowing quick comparisons without navigating away from the main view. However, it was not ideal for handling high volumes of buyers, as it required frequent interactions to browse through multiple matches, slowing down the process.
After collaborative discussions with product manager, engineers, and marketers, we chose Version 4 (Card View with Interactive Side Panel) for usability testing as it best balances among clarity, depth, and usability. This version enables quick buyer scanning, provides matched buyer company details in a side panel, allows users to pin buyers and add them to a master list, and clearly distinguishes between seller and buyer companies.
We invited 3 M&A advisors for usability testing, and here are some user quotes to guide the final iteration:
Iteration based on usability testing and feedback gathered from 3 advisors.
Final Design - AI Matched Buyers
Final Design: Matched buyer List
Listed project: Seller-side details
Final Design: Filter on Matched buyers page
AI Matching Setup
Comprehensive Buyer Profiles – The recommended list now includes detailed buyer information such as company type, M&A strategy all in a single view.
Advanced Filtering & Faster Prioritization – Sort buyers by company type, investment size, and M&A strategy to quickly identify best-fit matches, streamlining evaluation and decision-making.
Customizable Ranking – Users can fine-tune matching formulas and weighting factors, ensuring selections align with specific deal criteria and seller preferences.
I further iterated the matched buyer page, to help users quickly evaluate and prioritize the best-fit buyers with comprehensive profiles, advanced filtering, and real-time customizable ranking, ensuring faster and data-driven buyer shortlisting. Key Improvements are:
Ideation & Iteration: Matching Analysis
To design an effective Matching Analysis page of the matched buyer details , I explored four versions to enhance transparency, improve buyer evaluation, and streamline decision-making. Advisors needed an effective way to assess buyers, but their priorities varied—some preferred quick overviews for fast decision-making, while others required detailed financial and strategic insights. The challenge was to balance clarity, usability, and depth to meet all user needs.
Version 1: Interactive List View with Slide-In Buyer Insights
Pros: Improved Accessibility – Users can view detailed buyer insights without navigating away from the main list.
Cons: Split Focus – The simultaneous view of the list and slide-in panel can be distracting, making decision-making less efficient.
Version 2: Comprehensive Buyer Dashboard with Slide-In Panel
Pros: Visualized dashboard simplifies buyer evaluation with intuitive matching criteria, enhancing decision-making.
Cons: Lacks a structured ranking system, making it difficult to compare buyers quickly across key dimensions like financials, industry fit, and investment history.
Pros: Provides complete visibility into individual matches with a pivot feature for deeper analysis, enabling thorough buyer exploration.
Cons: Lacks contextual seller information, making it harder to see the bigger picture and less efficient for high-level decision-making.
Version 3: Full-Page Buyer Analysis with Pivot
Version 4: Simplified Buyer Cards for Rapid Scanning
Pros: Simple and intuitive layout for quick scanning of high-level buyer details.
Cons: Lacks proper grouping, leading to clutter and difficulty in comparing multiple buyers in large datasets.
Our team—product managers, engineers, designers, and marketers—analyzed four design versions for the AI Matching & Buyer Shortlisting interface. Our goal was to find a solution that enhanced decision-making efficiency, provided clear AI-driven insights, and balanced usability with depth. We finally chose Version 2 for usability testing as it provided the best balance of visual clarity, AI transparency, and multi-buyer comparison, allowing advisors to make faster decisions.
Iteration based on usability testing and feedback gathered from 3 advisors.
I further iterated the matching analysis page to help users gain more insights and greater control over high potential buyers with transparent match scoring, real-time ranking adjustments, and seamless quick actions, enabling efficient buyer evaluation and high-impact deal decisions.
Transparent Matching Score & Rationale – Provides detailed insights into match logic, helping advisors understand why a buyer is ranked highly, increasing confidence in AI recommendations.
Real-Time Buyer Assessment– Users can modify percentage priorities for key criteria (e.g., financials, industry fit) in real time, instantly updating matching score to evaluate how each buyer aligns with the seller’s needs.
Seamless Workflow with Quick Actions – Instantly send teasers, schedule follow-ups, or add buyers to a master list, reducing friction and improving deal momentum.
Final Design - Matching Analysis
Here shows the comprehensive user flow that streamline the M&A deal-sourcing process.
Data Gathering – Select a seller and input key criteria for accurate AI matching.
Smart Matching – Get top buyer recommendations with match scores.
Buyer Shortlisting – Review profiles, refine rankings, and save top matches.
Outreach – Connect with buyers via teasers and personalized engagement.
End-to-end Workflow Overview
Impacts
20% efficiency improved in deal sourcing
Smarter deal sourcing with AI insights
Improved client satisfaction with highly targeted deal matching
Reflections
Designing for complexity: M&A workflows involve multiple stakeholders with distinct needs, requiring a structured yet flexible system. I focused on mapping clear user flows, optimizing key interactions, and ensuring efficiency in a data-heavy environment.
Build for scalability and future growth: I approached design strategically, creating adaptable solutions that evolve with business needs and seamlessly integrate into industry workflows.
Bridge UX and business goals: I strengthened my ability to align UX decisions with business objectives, ensuring solutions not only enhance user experience but also deliver measurable value. Moving forward, I’ll continue designing with a strategic, impact-driven mindset that balances user needs with business success.
Thank You for Reading !
You've reached the end of this case study—thank you for taking the time to explore my work! I'm excited to share what I've been working on over the years.
Want to see more projects? Click the links below:
Next: AR Shopping Guide in Commercial Complexes
Previous: Redesigning HiveSpark AI 2.0-Marketing Process Management Platform